Reason and background
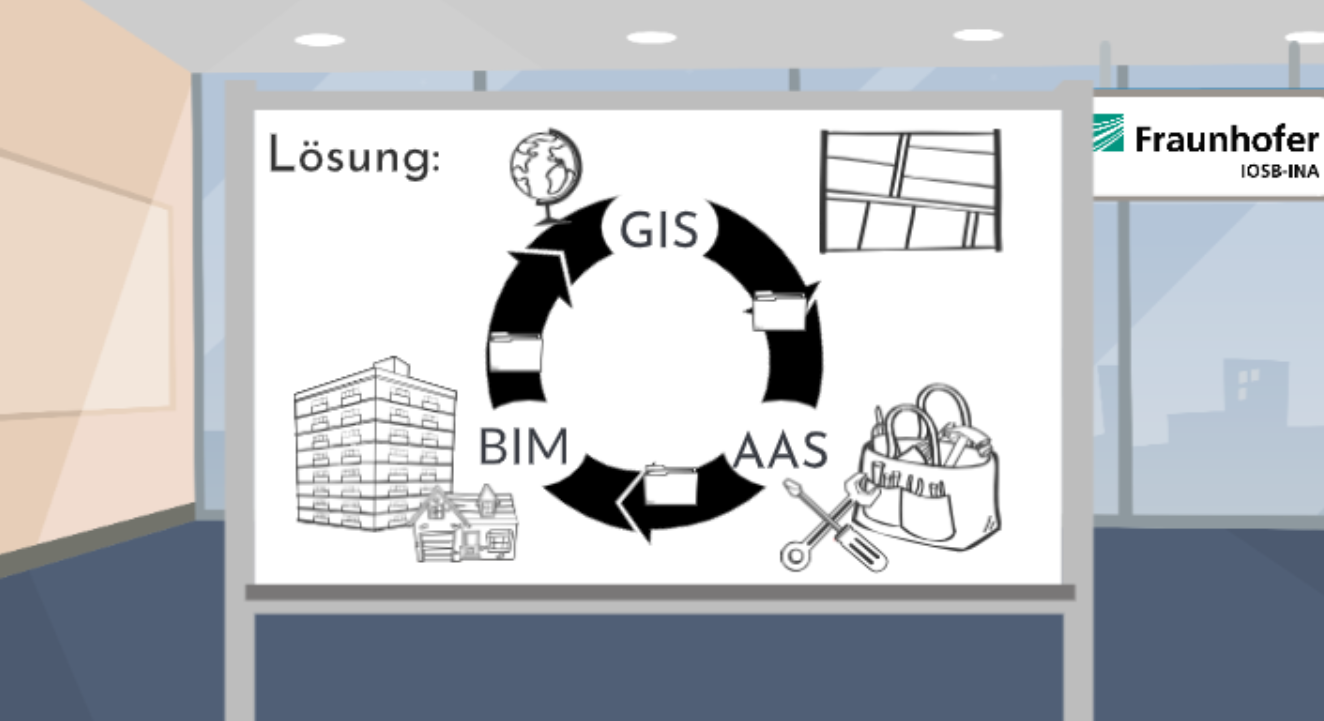
The energy performance of buildings is playing an increasingly important role for building owners, operators, local authorities and residents, not least due to rising energy prices. With the introduction of the Building Energy Act in 2020, public interest is focussing in particular on the energy-efficient refurbishment of existing buildings.
At the same time, cities and local authorities are becoming increasingly interested in providing digital services and information. If the information is linked to a 3D visualisation, this is also referred to as a digital twin of the city. However, there are usually major deficits in the level of detail, completeness and consistency of the data. Efforts are being made to standardise data management (neighbourhood level: CityGML, XPlanung, building level: BIM/IFC, facility level: Administration Shell (AAS)), but these are still not used and linked enough in practice. The energy consumption of buildings has so far been relatively complex and laborious to record and has therefore only been processed on a large scale in individual cases. The realisation of a "digital energy twin", which provides an overview of the energy consumption of individual buildings and entire neighbourhoods in a city, is currently still coming up against limits in terms of data collection, data exchange and data protection.